The uniform distribution reigns supreme as a champion of equality. Imagine rolling a fair six-sided die – each face, from one to six, has an equal chance of landing face-up. This scenario captures the essence of the uniform distribution: every possible outcome has the same probability. This article delves into the world of uniformity, providing diverse examples, unveiling its applications, and equipping you with the knowledge to recognize and interpret this fundamental concept.
The Essence of Equality
Picture a dartboard divided evenly into ten sections. Throwing a dart at random, each section offers the same probability of being hit – that’s the spirit of the uniform distribution. Mathematically, the probability of any single outcome within a defined range is constant. This characteristic makes the uniform distribution a valuable tool in various fields, where chance and randomness play a role.
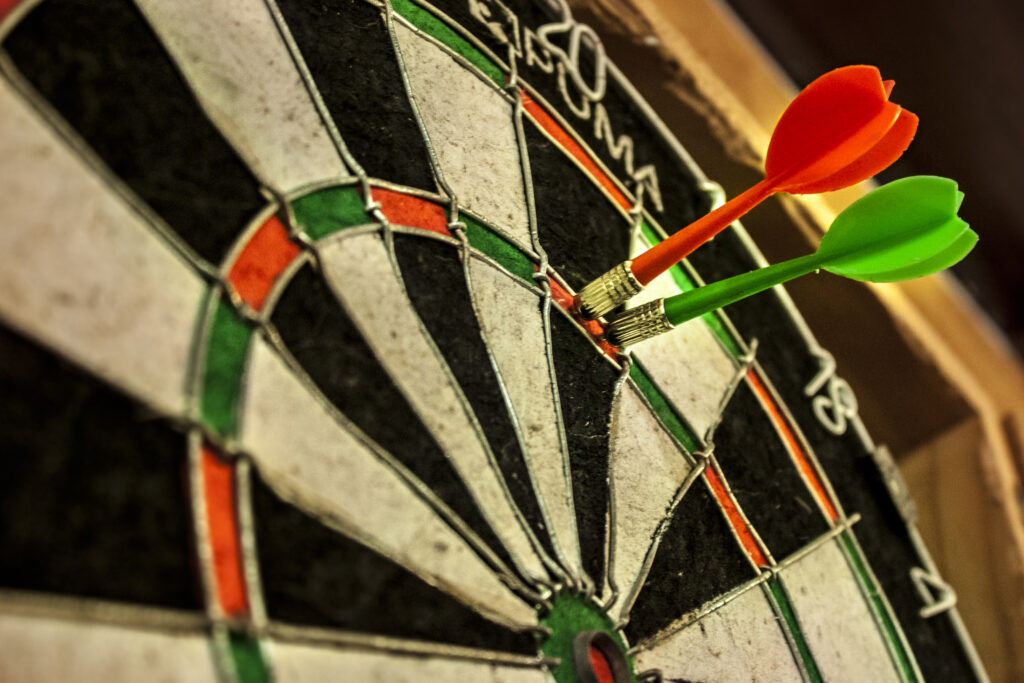
Where Uniformity Rules Across Disciplines
The uniform distribution manifests across diverse arenas:
- Games of chance: Rolling dice, shuffling cards, or spinning a roulette wheel – each outcome has an equal probability under the assumption of fairness.
- Random sampling: Selecting subjects for a study from a population, picking customers for a survey, or choosing songs for a playlist often involve random selection, often modeled by the uniform distribution.
- Monte Carlo simulations: Simulating complex systems with various random elements often relies on the uniform distribution to generate random values within defined ranges.
- Cryptography: Generating random encryption keys often utilizes the uniform distribution to ensure unpredictability.
The Applications of Uniformity
The uniform distribution isn’t just about chance and games; it serves various purposes:
- Evaluating statistical tests: By generating data following a uniform distribution, we can assess the performance of statistical tests under controlled conditions.
- Estimating probabilities: In situations where information is limited, the uniform distribution can provide a starting point for estimating probabilities before gathering more data.
- Modeling uncertainties: When factors affecting an outcome are unknown or unpredictable, the uniform distribution can represent a range of possibilities.
Visualizing Uniformity: Tools for Clarity
Visualizing the uniform distribution is straightforward:
- Probability density function (PDF): This graph is a horizontal line across the defined range of possible outcomes, representing equal probabilities for all values.
- Cumulative distribution function (CDF): This graph increases linearly from 0 to 1 as the values progress through the range, depicting the probability of encountering a value less than or equal to a specific point.
Beyond the Flat Line: Considerations and Limitations
Remember, the uniform distribution assumes equal probabilities within a defined range. This means:
- Limited to specific ranges: You can’t use it for outcomes with infinite possibilities like real numbers.
- Potential misinterpretations: In real-world scenarios, true randomness might not be perfect, leading to deviations from the ideal uniform distribution.
Leave a Reply